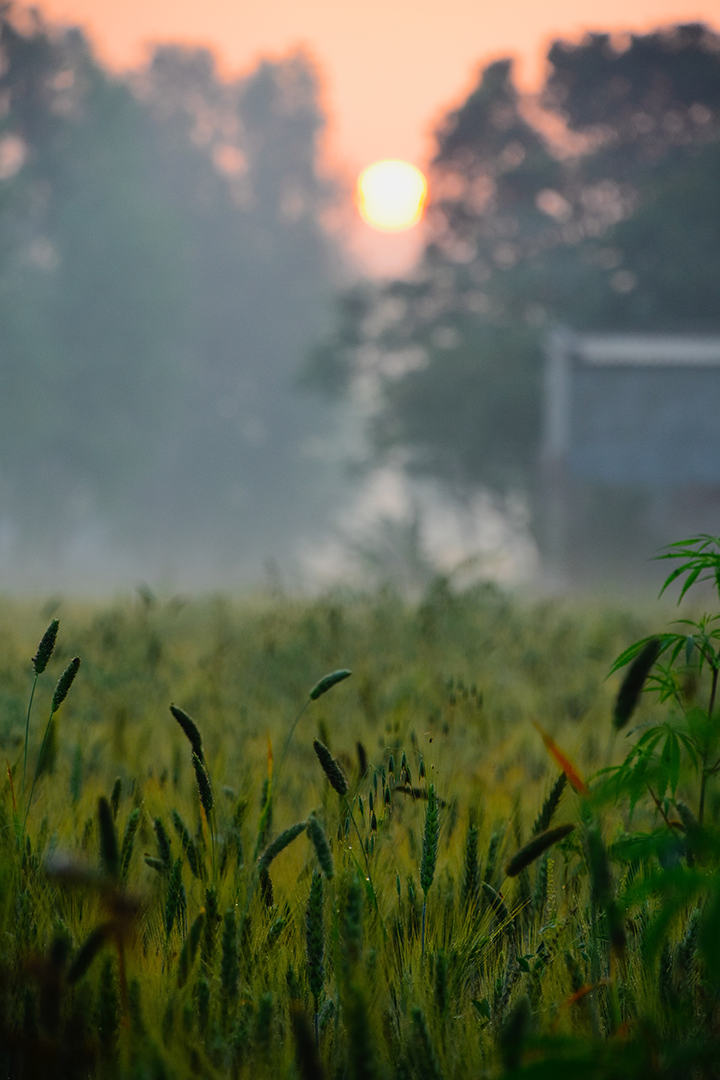
Farmer-Centric Data Governance Assessment: A New Paradigm For LMICs
Why a New User-Centric Paradigm is Imperative
The benefits of digital agriculture are prolific. Digitization makes it possible to trace supply chains, treat plants precisely, or obtain data on the status of soil. Digitization holds great promise to modernize the agriculture sector, including by increasing access to finance and helping to combat climate challenges. Data-led efforts are critical, particularly when a lack of access to reliable data threatens humanity. The value of data is unquestionable.
While much data is generated by farmers, it generally is owned and controlled by others. Farmers rely on external resources, like weather data and proprietary tools, that provide specific advisories inferred from data. Yet farmers increasingly lose control over their data and the benefits derived from it. Other dangers exist, with intermediation, opaque data value chains, and dominant market mechanisms. The rapidly increasing data extraction is of great concern, especially in light of current data governance practices, insufficient regulatory guidance, and other capacity restraints. As a result, some farmers are becoming reluctant to share data, also placing them in the position of having to forgo access to beneficial services.
Agriculture’s potential is limited by dominant monopolies and asymmetries of power and information (i.e., when some groups have access to more information than others). The current data economy — certainly at the level of global, big data initiatives — is defined by a paradigm of extraction and a lack of consideration of equity, whereby individuals and communities go unrecognized beyond their use as data points and producers. This has implications on the success of development programs, particularly for economic inclusion and growth.
Reflecting on these issues, many questions arise. For example:
- Who controls the data and what does farm data ownership mean?
- Is it in the interests of smallholders to provide data to firms that share or sell the data with other agribusinesses, banks, or insurers?
- Would it be preferable to retain control and make decisions about how data is used?
- What level of participation should we expect from farmers and farmer groups in data governance?
Why User-Centric Data Governance?
How data is governed informs what products, services, and insights are used and shapes program strategy. Empowering farmers with more control over their data is critical to improve and protect their livelihoods.
User-centric approaches to data governance place farmers, as beneficiaries, at the center of data initiatives which reduces the negative effects of centralized power. User-centric models grant farmers and their communities greater agency over their inputs and outputs and meaningful participation shaped by their immediate needs.
Models like data collaboratives, data commons, data cooperatives, and decentralized data fiduciaries hold the potential to strengthen the position of farmers as equals in future developments. These evolving approaches transition control of data and safeguards against privacy invasion, data misuse, opacity, and other harms. Models can also generate better data sharing opportunities, counter data fragmentation, increase data quality, and identify avenues for innovation.
Farmer-centric, participatory models hold the potential to shift the hegemonic paradigm toward long-term, sustainable, equitable, trustworthy, and more successful solutions. Greater participation in the creation and implementation of data governance can bring about greater societal and economic equity and contribute towards increased public confidence in the use of data.
Given the momentum of digital agriculture and investment strategies, it is crucial that equitable models of data governance are placed within the ecosystem as soon as possible. There is a pressing need to unpack these practices, learn about their challenges, and think about measuring their impact. This study attempts to move beyond the theory covered by others to explore the practical implementation in LMICs, their elements of success, with a focus on agriculture.
Who Benefits from Farmer-Centric Data Governance?
- Farmers gain more control and agency over their data, more equality, meaningful participation and representation, bargaining power, alignment with interest, and access to new markets and opportunities.
- Agribusiness, tech providers, governments and development organizations, benefit, among others, from:
- better and more consistent, reliable, recent, higher quality data, enhanced data access and availability, greater data sharing opportunities, enhanced data management, and decreased data fragmentation;
- greater efficiency and productivity, (public) service design and delivery, decision making, situational awareness and response;
- improved mediation and formalized relationships, communication, transparency, meaningful feedback and nurtured trust; and,
- improved reputation, public relations, legal and privacy compliance, responsibility and meaningful corporate social responsibility;
- increased knowledge creation and transfer, research opportunities, value creation and new avenues for innovation.
About the Study
The objective of this study is to showcase opportunities of user-centric models and the enabling environment needed for implementation. In partnership with USAID, BMGF, and DAI, the study conducted by Development Gateway and Athena Infonomics aims to:
- raise awareness around the current data economy and implications of the status quo;
- identify user-centric data governance models and mechanisms, particularly in LMICs;
- place farmer-centric data governance approaches on the radar of primary stakeholders;
- demonstrate purpose, value, and benefits (as well as challenges) of these practices; and
- identify if support for implementation is needed, for whom, and in what form.
Below we introduce a primer of user-centric, participatory data governance. These practices are not exclusive or exhaustive and often seek different objectives. You might already employ a number of these approaches.
Data collaboratives promote collaboration between diverse organizations, harness their collective capacities and insights and enhance access to wider institutional data which would be outside their purview. Data collaboratives provide organizations with an option to decide rules of data exchange. This also provides a way of gaining trust and confidence between stakeholders. The model can be used as a term to describe initiatives where private sector data is combined and shared with a third party who manages access to it, usually to make proprietary or siloed data available to inform research or public sector decisions. Data collaboratives need responsible, tenable data stewards to empower members or the general public.
Data marketplaces are platforms where data providers (sellers) and data consumers (buyers) can meet, match, and trade their respective data assets and requirements. Marketplaces are emerging as new intermediaries and increasingly play a vital role in the data economy. Acting as an intermediary, they may increase willingness to share data. Farmers may also have access to aggregated platform data on key market trends derived from data supplied by its users. Marketplaces empower smallholders, especially in LMICs, by generating and sharing data on prices, transaction history, and demand. Data marketplaces can assist in overcoming economic and environmental challenges, optimize productivity, and cut costs.
Data commons pool and share data as a resource, with a high degree of community ownership and leadership. This approach can address power imbalances by democratizing access to and availability of data. They can be created with a variety of data and governance structures. A prerequisite is that they be stewarded responsibly (for which many refer to Ostrom’s principles). In science communities, research data is often pooled to increase the impact of data held by individuals. The discourse revolves around the learnings of open access and application of new forms of management.
Data fiduciaries are a type of steward acting as an intermediary that manages access to data between individuals and data collectors based on a duty of care. A data fiduciary can be used to create a trusted environment between various stakeholders and assist in overcoming power imbalances. Mediation of data relationships through a data fiduciary allows for a representative control model which enables individuals to have more control over how data is used and shared.
Indigenous data governance shifts access and control of data away from governments and others directly to Indigenous Peoples. Considering how withholding information has been used as a vector of control, these approaches illustrate how important data sovereignty can be to self-determination. Here, data stewardship entails governance on behalf of (and by a community in) the entire data lifecycle. The mainstream open data and FAIR data principles discourse have been faulted for ignoring historical contexts and power differentials and propose safeguards in the CARE Principles.
Data cooperatives are voluntary communal pooling by individuals of their personal data for mutual economic, social and cultural benefit, and aspirations of a group through a united, jointly-owned, and democratically controlled autonomous association. The model works when stakeholders can be given an equal opportunity in organization and management and have a collective interest. Data cooperatives grant farmers more control over their data; they can manage, curate, and protect access to their data. At the same time, it offers an innovative approach to foster direct engagement and represent interests. Cooperatives come in many forms as they evolve out of the needs of their members.
General Actionable Principles
- User-centric models should be integrated into digital agriculture technology programs. These models have immense potential to shift the current paradigms of information imbalances to benefit farmers, communities, and societies. User-centric models can empower individuals to gain more control and ownership over their data, create individual or collective agency and negotiation power, and protect against data misuse.
- Farmer-centric data governance approaches pursue more consistent and higher quality data sharing, interoperability, and defragmentation. Its impact is dependent on whether design, deployment, and implementation are made collaboratively, building on a foundation of trust. This creates true incentives and can overcome the increasing unwillingness of farmers to share data.
- Meaningful participation must strongly tie inputs and outputs of farmers to data governance. Farmers and their communities should have visibility into practices and avenues for voting, inquiry, redress, and rectification. Feedback should contribute to decision-making and strategic direction. This requires close monitoring to ensure not only that these requirements are met but also sustained throughout the entire engagement lifecycle.
- Data stewards play a crucial role as trusted intermediaries between farmers, data collectors, and data generators. Including a steward enables more access to data pooled by farmers, data that can be shared for broader social benefit, and for a better bargaining position. More research is needed to conclude exact fiduciary relationships and roles of data stewardship.
- Trust needs to be fostered. The need to establish and maintain trust is foundational for success in any engagement, if digital AgTech and data are to transform agri-food networks. Efforts that integrate data and data collection products must clearly explain what activities are being undertaken, their benefits, privacy measures, and the process as to how questions and concerns are addressed and resolved; all of this needs to be included not only at the start of an engagement, but with a plan to maintain these conversations and services throughout.
- Center local context and culture to determine outcomes, implications, and impact when considering models. Farmers are not homogenous, and their needs vary even on a local level. Women and Indigenous Communities often lack representation and agency. User-centric models may very well help, but should not be rolled out in a generic fashion. Context helps define and decide which approaches are most appropriate. Even the best-fit model can bring harm if it is seen to compete with or supplant existing and trusted community dynamics.
- Prioritize existing practices in the communal governance and their research in LMICs, rather than approaches that strongly reflect Anglophone, Western concepts. Data and information sharing in agriculture often happens via less formal constructs. Language barriers constrict examining resources on experiences outside the expected avenues. Communities often know best how to organize and govern resources. Research and implementation need to follow systematic observation of diverse communities with different vantage points in multiple case studies.
- User-centric models are not a panacea ‘one-size-fits all’ solution. Data governance applications tend to be hybrid, iterative, and adaptive. The practical implementation is a lot more ambiguous and variable than theory often suggests. A meaningful farmer-centric data ecosystem can only be built on a range of models and mechanisms. Multi-level governance is an important component of successful engagement. It is important not to get carried away by any hype, instead focus on identifying exactly what is needed.
- More research is needed to identify training and capacity building requirements and financial sustainability. The data governance models showcased here are the most prominent examples in an expanding field of opportunities with a newly emerging set of organizations specialized in different avenues of data governance. There is a need to develop data governance skills training aimed at farmers and the development community to assess and capitalize on user-centric data governance opportunities.
Share
Recent Posts
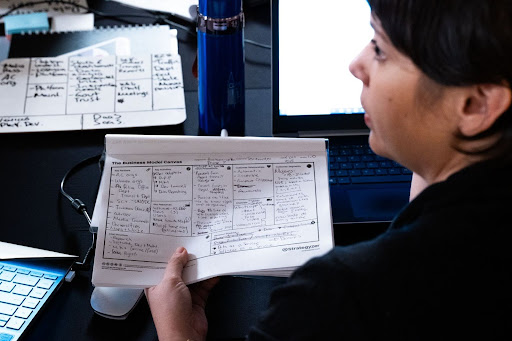
DG Developing Open Source AI Tool to Help with the Fight against Corruption
We’re excited to announce our forthcoming open-source AI tool to support anti-corruption work. The tool, which we hope to register as a Digital Public Good, will be used to extract text from complicated contracts and support anti-corruption efforts by increasing accessibility of and transparency around hard-to-access information.
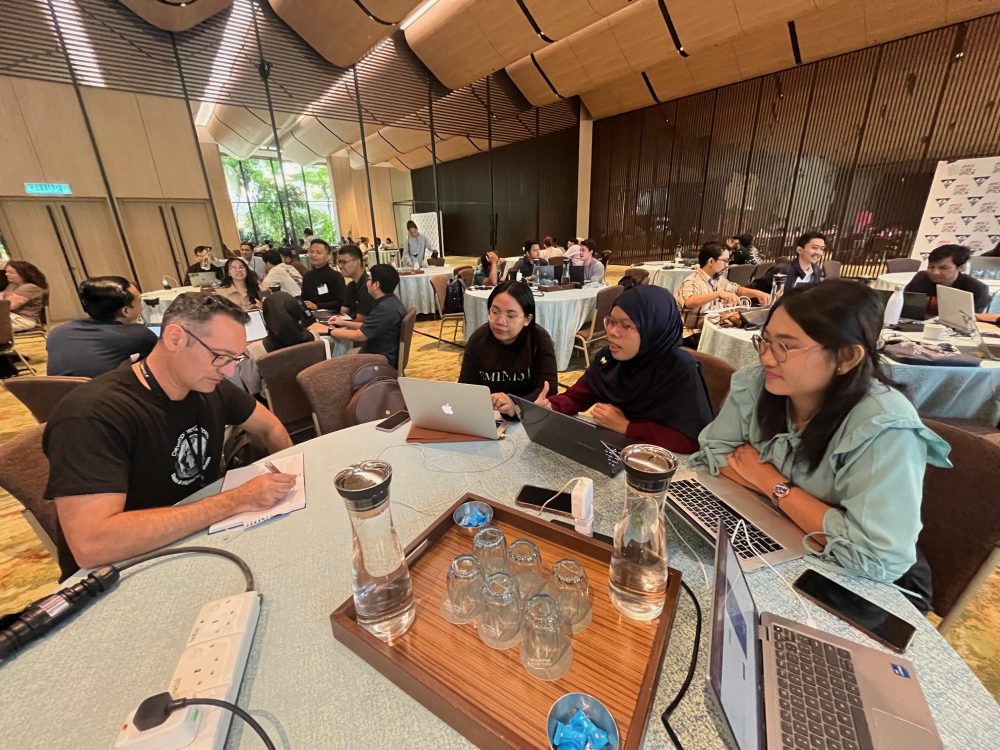
Three “Hacks” in Advancing Anti-Corruption Work To Strengthen Accountability
Since partnering with Accountability Lab (AL) on HackCorruption in 2023, staff from Development Gateway: An IREX Venture (DG) and AL have mentored teams from the hackathons hosted by the project, which is aimed at leveraging innovative digital tools to identify and fight corruption. After serving as the mentorship team for hackathon winners, DGers Gabriel Inchauspe and Kelley Sams, along with AL’s David Sada have identified three hacks in mentoring anti-corruption change-makers and ultimately, furthering transparency and accountability through the creation and use of digital tools.
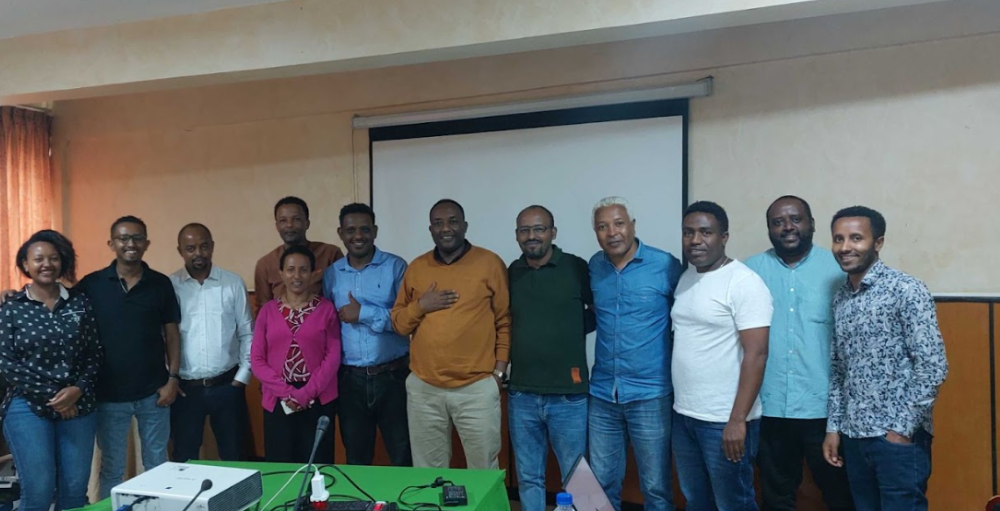
aLIVE Program Reaches Milestone: Livestock Data Standards Endorsed by Ethiopia’s Ministry of Agriculture
In early 2024, the "a Livestock Information Vision Ethiopia" (aLIVE) governing committee endorsed a comprehensive set of standards to guide the collection, storage, and maintenance of livestock data in Ethiopia (i.e., a data standard). The data standard specifically focuses on standardizing data on cattle, sheep, goats, and camels in the country. The National Livestock Data Standard document contains standardized data sets for national animal data recording, animal disease, diagnosis, treatment, vaccination recording, animal events recording, location, and other additional attributes.