What does Data Interoperability Require in Practice?
A few months ago, Development Gateway (DG) and our partner Athena Infonomics (AI) began the groundwork to improve the interoperability, analysis and – ultimately – use of agriculture and nutrition data in Cambodia and Nepal. Under the mSTAR project funded by USAID, DG and AI set out to understand the underlying structure of the data currently being collected and managed by Feed the Future implementers, and how to best support them to open up and share their data through digital tools and best practices. With the goal of accelerating data-driven agriculture development, DG and AI are supporting these partners and the USAID Missions in Cambodia (USAID/Cambodia) and Nepal (USAID/Nepal) to increase the availability of relevant data for research, analysis, and learning across stakeholders.
After assessing the challenges and opportunities for leveraging open agriculture and nutrition data in each country, we reviewed sample datasets to define the key components of a common data structure capable of improving interoperability across datasets and implementers. We also explored options for deploying a data repository – a solution for importing, storing, and retrieving digital content – to improve data sharing. Three main takeaways emerged, which we believe are relevant not just for data managers in Cambodia and Nepal but also for other contexts and sectors:
1. It is possible to achieve a common data structure – provided some common-sense principles are followed.
Our analysis of sample datasets from 13 USAID-funded research groups and implementing partners in both countries revealed similarities in the data they collect. But, due to differences in the way datasets are structured and variables are defined, stakeholders cannot currently make use of each others’ data – including socioeconomic and experimental data on agricultural practices and nutritional statuses – to inform their own programming. In order to help stakeholders discover and reuse relevant data, a number of interoperability standards and principles must be followed. This includes adopting frameworks that help ensure that datasets are easy to search for based on their content and any user can interpret and use their content.
The former can be achieved when data publishers capture important metadata information on the project and dataset, which includes defining and detailing elements like scope, data collection methodology, data availability, and terms of use. Adopting a standard ontology or vocabulary – a formal naming scheme, such as AGROVOC, that defines the types and properties of variables – during data collection and reporting helps improve future usability of a dataset. Similarly, to ease integration with other applications and repositories, stakeholders should agree on naming schemes for country-specific variables (e.g. administrative boundaries like provinces or municipalities), standard units of measurement (e.g. area, length, weight, etc.), and following basic structural hygiene standards (e.g. keeping data belonging to the same dataset within a single file, and using clear column and row names and codes for missing data). Finally, we suggest that all dataset owners create corresponding codebooks documenting all of these elements of the metadata schema.
2. Existing storage solutions meet stakeholder needs.
Our analysis also explored options for storing partners’ datasets – looking broadly from building a custom repository to deploying one of hundreds of existing digital data repositories. Many of these meet basic data management and storage needs, and several free or nearly free options have advanced functionalities that meet best practices for uploading, storing, and sharing data.
Drawing on the FAIR principles and guidelines by the Data Curation Centre and Data Seal of Approval, we considered critical features for any data storage solution, including the ability to upload and share various data types, assign unique identifiers, restrict access to certain data, apply reuse licenses, be discoverable by search engines, and link to APIs. When existing storage options meet user needs, we do not recommend investing the time and significant resources required for designing and building a new repository. In this case of USAID Cambodia and Nepal stakeholders, a solution like Harvard’s Dataverse also offers relevant, advanced features that would allow the Missions to further customize the tool – including rich and granular metadata fields; advanced search functions; customized use metrics; auto-generated “preservation” file formats; built-on integrations for data analysis and visualization; and links to other data catalogs.
3. Strong, central administration is crucial for a sustainable data repository.
Finally, designing a practical implementation strategy requires an effective governance structure, capable of and adept at managing the repository. In defining clear oversight and support tasks, you must consider organizational roles and capacities. For USAID/Cambodia and USAID/Nepal to facilitate smooth set up and implementation, we recommend they determine a set of procedures and guidelines for partners and researchers who will be uploading data, and agree on roles and responsibilities with each stakeholder group.
To ensure compliance, all data managers should agree on data quality, sharing, and usability protocols prior to the rollout of any repository, including sharing all data underlying research publications, establishing timelines for data uploads (e.g. at the time of publication for researchers and within one year of collection for implementing partners), handling sensitive information, and promoting discoverability through linkages with other relevant repositories. . Additionally, USAID should take on additional data preparation and curation tasks such as supporting initial repository setup, tracking data submissions, approving and publishing data, and assisting partners with adopting new standards and processes for dataset preparation and upload. DG and AI recommend the Missions prioritize stakeholder buy-in for these new roles and responsibilities, and convene annual in-country open data working groups to sustain stakeholder interest and regularly address issues as they arise.
Together, these data management recommendations provide actionable strategies for migrating to a common data structure and implementing a practical data storage solution. Recognizing the complexities and nuances involved in managing data and designing country-appropriate systems, our next steps aim to build stakeholder buy-in and capacity to make the most of their data – through in-country workshops and targeted technical assistance to users across both Cambodia and Nepal to improve interoperability, knowledge sharing, and food security outcomes.
Enjoy this post and our first piece about DG’s work supporting FHI 360’s Mobile Solutions Technical Assistance and Research (mSTAR) project? Stay tuned for updates as we continue progressing towards strengthening data interoperability, knowledge sharing, and food security outcomes in Cambodia and Nepal.
Thumbnail image: Kathryn Alexander, the Feed the Future demonstration plots at CE-SAIN/RUA in Phnom Penh
Share This Post
Related from our library
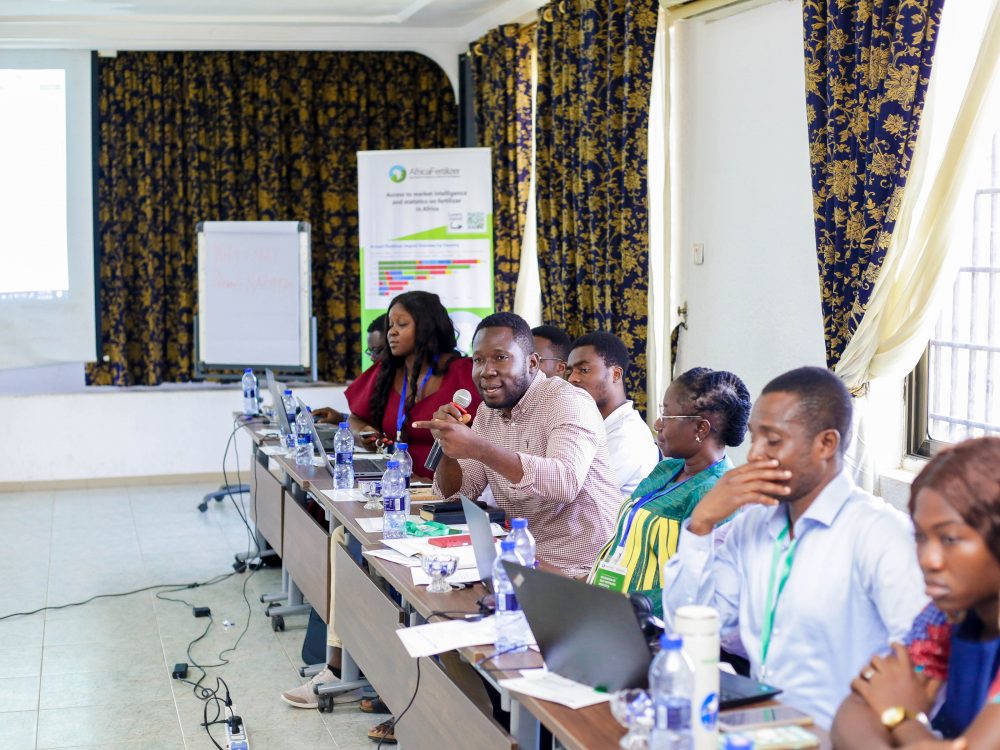
Case Study: Fostering Sustainable Agriculture through Data-Driven Collaboration and Partnership: Ethiopia, Mozambique, and Nigeria
Through DG’s Visualizing Insights on Fertilizer for African Agriculture (VIFAA) program, we recently published a case study titled “Fostering Sustainable Agriculture through Data-Driven Collaboration and Partnership: Ethiopia, Mozambique, and Nigeria.” It dives deep into how the VIFAA program has impacted the fertilizer data and markets in Ethiopia, Mozambique, and Nigeria. In this blog, we explore the overall impact that the VIFAA program is making, why the program was needed, and offer some key highlights from the case study.
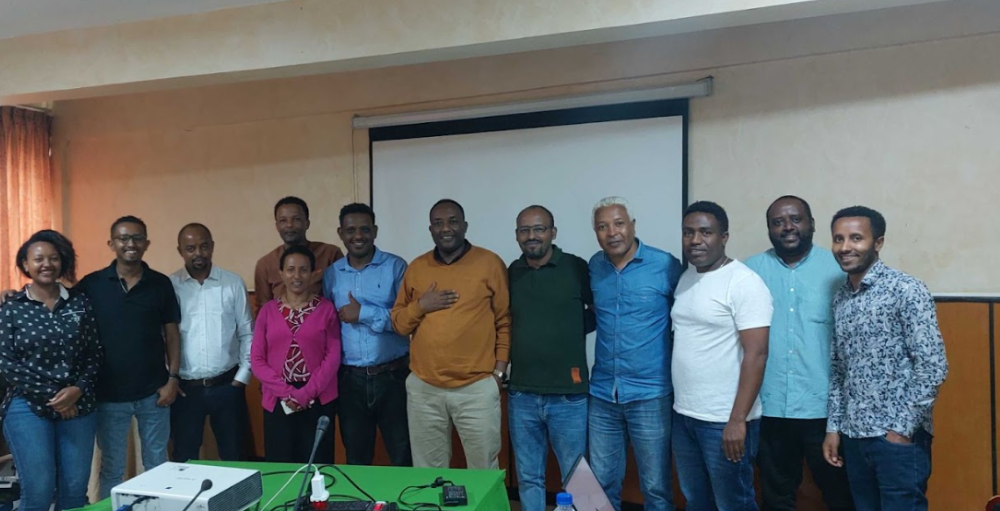
aLIVE Program Reaches Milestone: Livestock Data Standards Endorsed by Ethiopia’s Ministry of Agriculture
In early 2024, the "a Livestock Information Vision Ethiopia" (aLIVE) governing committee endorsed a comprehensive set of standards to guide the collection, storage, and maintenance of livestock data in Ethiopia (i.e., a data standard). The data standard specifically focuses on standardizing data on cattle, sheep, goats, and camels in the country. The National Livestock Data Standard document contains standardized data sets for national animal data recording, animal disease, diagnosis, treatment, vaccination recording, animal events recording, location, and other additional attributes.
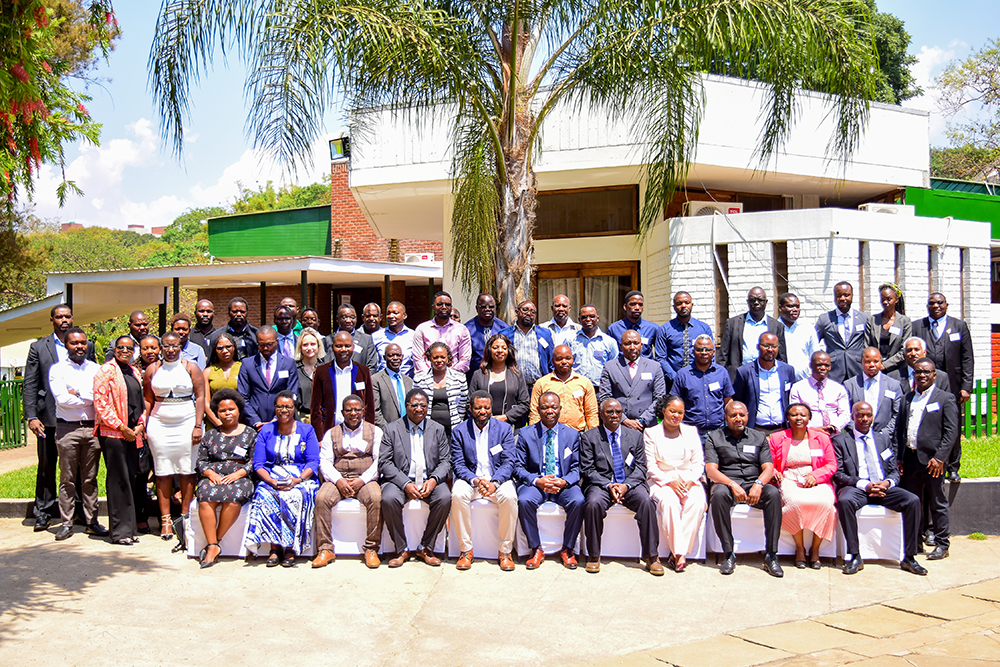
Unlocking the potential of digital public infrastructure for climate data and agriculture: Malawi
DG’s DAS Program recently attended an event on creating a national digital public infrastructure (DPI) in Malawi in order to increase the impact of climate data to combat current and future agricultural issues caused by climate change. In this blog, we reflect on three insights on DPIs that were revealed during the event discussion.