Artificial Intelligence: A Silver Bullet or Scrap Metal for Global Development?
When someone mentions artificial intelligence (AI), it’s easy to conjure up two conflicting images: the first, killer robots whizzing past, replacing human jobs, daily tasks, and social interactions in a post-apocalyptic world; the second, a C-3PO-esque personality revolutionizing our health and food systems. Pondering this, we are also inclined to explore the question, where does global development fit in within this futuristic, Star Wars-inspired universe?
Our recent work collaborating with AidData to design a machine learning model predicting humanitarian trends, and our participation in an Open Data & Artificial Intelligence Roundtable at the Open Gov Hub, has reinforced for us that AI isn’t a “silver bullet” for global development. However, when used ethically, it can be (another) tool in the toolbox for tackling persistent global challenges.
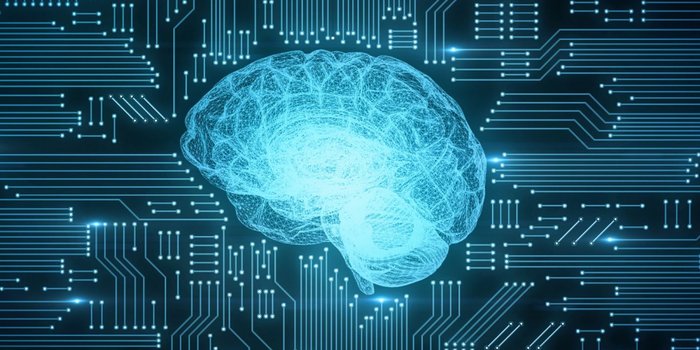
So What’s the Skinny on AI?
AI is the training of computers to automate decisions in order to simulate human-like decision making, while machine learning (ML) is a subset of AI that teaches computers to recognize patterns in data, using those patterns to make future predictions. Already, both are rife with possibilities for better development outcomes. AI is currently being used as a tool to improve health service delivery, implemented through mobile phones to make health screening more widely available for patients in rural, often underserved areas.
However, the downside is that AI has come under pressure for perpetuating racial discrimination in facial recognition technology. The same technology has also appeared in some countries as a tool to identify (and jail) political dissidents. While these varying outcomes are not unique to AI (plenty of repressive regimes have targeted other digital tools to perpetuate discrimination), this does mean that international actors hold significant ethical responsibility as they increasingly turn to AI to enhance programs on everything from agricultural value chains to health and wellness.
Factors to Consider
Pick your problems.
Not all problems can, or should, be solved by AI. Why? Because the use of AI is heavily shaped by the context surrounding the problem: the culture and processes already in existence, and the cost-benefit analysis of redesigning the culture so that AI can be used effectively. For example, for a health program operating in a rural area where community relationships are highly valued, AI could speed up the time needed to read x-rays, but it cannot effectively replace the program’s human elements – for example, the relationship between local health extension workers and community members that drives preventive care, such as motivation to get x-rays.
The ideal mix is a blend of humans and machines working together, resulting in the slow, time-adjusted reorganization of work that reflects which tasks can be completed by a machine, and which should be completed by a human.
AI requires investment.
AI is not like a microwave – you can’t “set and forget it.” AI models are affected by “concept drift,” meaning they have to be routinely re-calibrated with new data so that they continue to produce “correct” decisions. At DG, we learned this first hand in developing the Autogeocoder tool which uses machine learning to read through project documents and pinpoint specific activity locations. We continually updated our model to address questions such as, what happens when a project’s activities occur in multiple locations at once? Or when the document mentions other, unrelated locations such as the implementing partner’s mailing address? The Autogeocoder model needed to be trained, consistently and by many users over time, to become effective at making these important distinctions.
Mind the accountability/privacy gap.
AI can help increase the quality of public service delivery, but it can also exacerbate a lack of transparency around decision making. As with the open data world, basic principles and guidelines do exist that outline how algorithms can become accountable. Additionally, there is a developing methodology that breaks down how algorithms come to make their decisions, which can help identify bias. In the push for transparency in AI practices, France is leading the way by making public all algorithms its government uses.
Closely related to transparency concerns is the need to protect individual data, Vulnerable groups remain at risk in digital development spaces – highlighting just how essential it is that safety-oriented, post-colonial, and gender-focused concerns frame how, when, and why individual data is used to feed AI models.
Some Best Practices for Using AI
AI is already showing incredible ability to answer complex development questions, so it’s not all doom and gloom! As a result, we’ve identified three approaches that can help anchor AI models as solutions-oriented tools to tackle global challenges:
Talk to people.
Conduct an assessment before you build the AI model to gain a basic idea of the availability, quality, and timeliness of data that will feed your model. We developed CALM as a method to navigate assessing data ecosystems, to then build tools and processes that address the most pressing decisions.
Remember partnerships.
Consensus building through human partnerships remains essential, from accessing the data needed to teach the model, to ensuring that the model ultimately solves the problem raised by citizens/end users.
Draw parallels.
The medical industry has frequently balanced open data, informed consent, and restricted access, offering examples of how the data for development community could also weigh innovation with individual rights.
Where do we go next?
There is no “one size fits all” answer to solving global development challenges. Ultimately, the power of AI rests with the humans behind it, and hinges on their ability to connect AI to other digital and analog tools – that, collectively, can address complex problems. Effective use of AI will require proponents and skeptics alike to proceed both cautiously and with an open mind, to ensure that our analog weaknesses don’t become our digital ones.
Resources to Dive Deeper
- A People’s Guide to AI by Mimi Onuorah and Diana Nucera
- Reflecting the Past, Shaping the Future: Making AI Work for International Development by the Center for Digital Development at USAID
- The Key Definitions of Artificial Intelligence (AI) That Explain Its Importance by Bernard Marr for Forbes
- Interactive Database of Government Policies Addressing AI by Nesta
Image credit: Mike MacKenzie, (CC-BY-2.0)
Share This Post
Related from our library
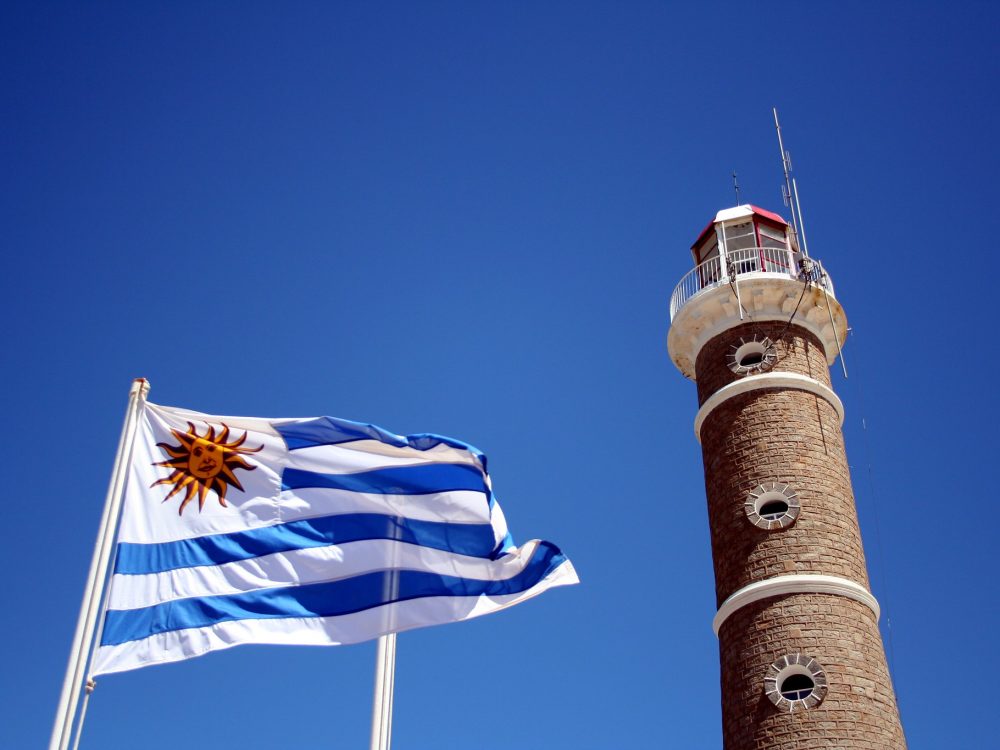
How Increasing Trust Can Help to Deliver the 2030 Agenda
The Festival De Datos is here, marking a pivotal moment to assess our journey with data for development. At DG, we've championed leveraging data and tech for a more equitable, sustainable world. But to fulfill this vision, we need to push for a fair data future and establish a culture of trust and cooperation in data use.
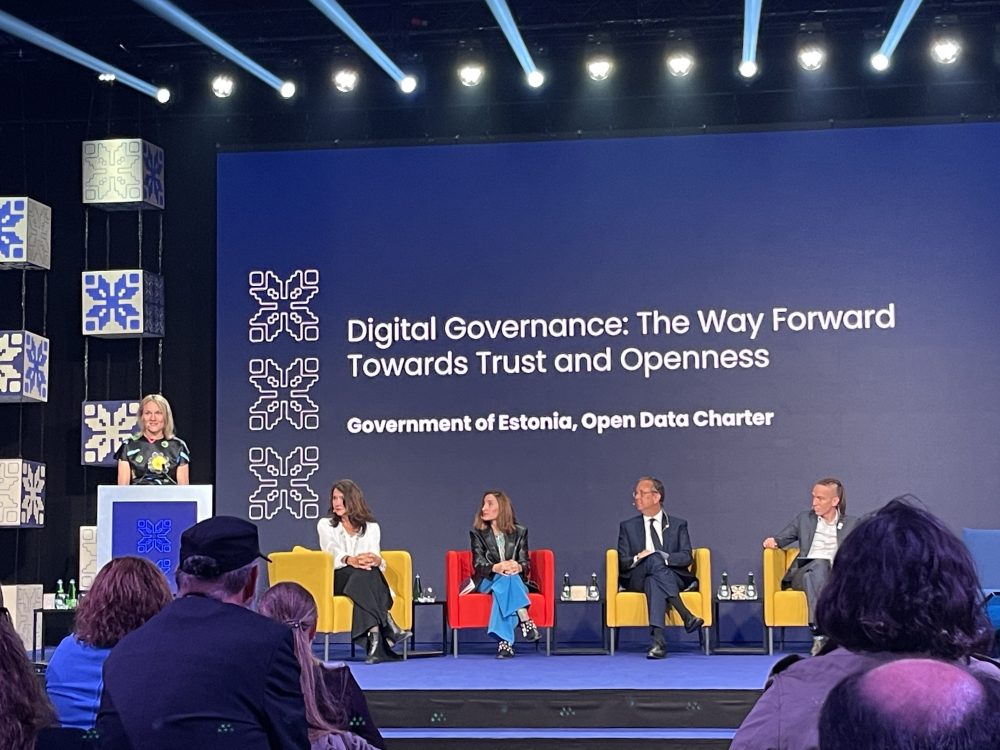
Democratizing Digital or Digitizing Democracy?
The 2023 OGP Summit in Tallinn, Estonia featured a number of discussions centered on open government in the digital age. While the use of digital tools in government is far from a new idea, the COVID-19 pandemic spurred a rapid expansion of this practice, with leaders quickly adapting to remote environments through digitizing government processes
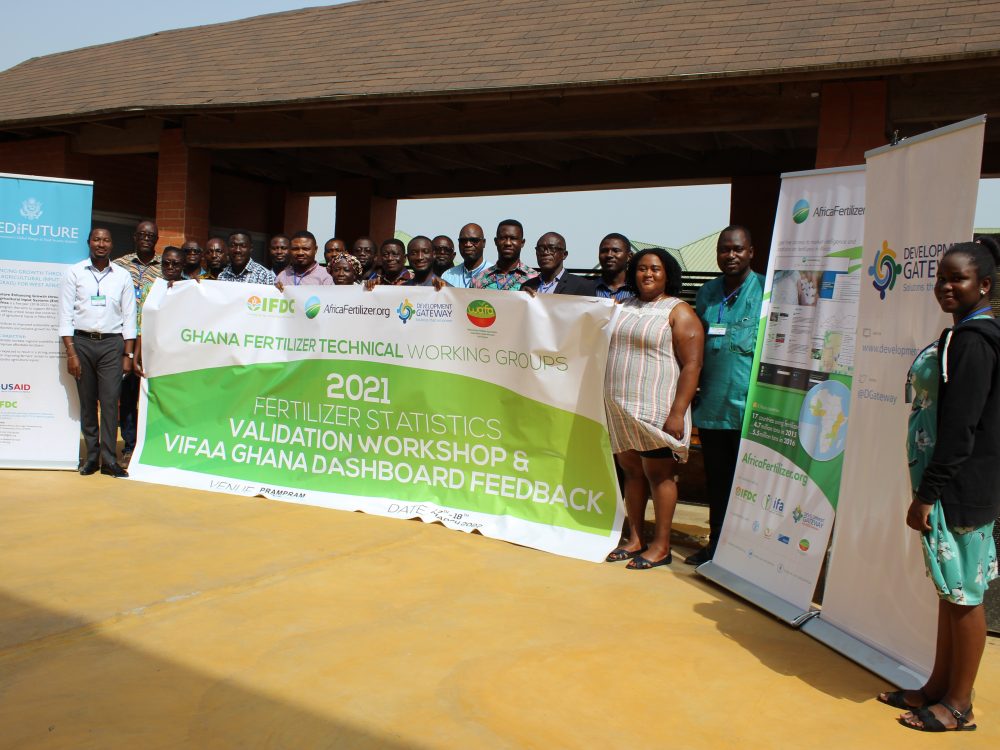
Fertilizer Technical Working Groups Provide Key Insights into Africa’s Fertilizer Sector
From June 2021 to September 2022, Development Gateway: An IREX Venture’s (DG’s) Visualizing Insights on Fertilizer for African Agriculture (VIFAA) program convened 12 Fertilizer Technical Working Groups in 14 countries which have yielded essential information on Africa’s fertilizer sector, including insights on how geopolitical events have impacted the fertilizer sector and what is needed to mitigate resulting threats to food security throughout Africa.