Posts categorized Explainer
Page 2
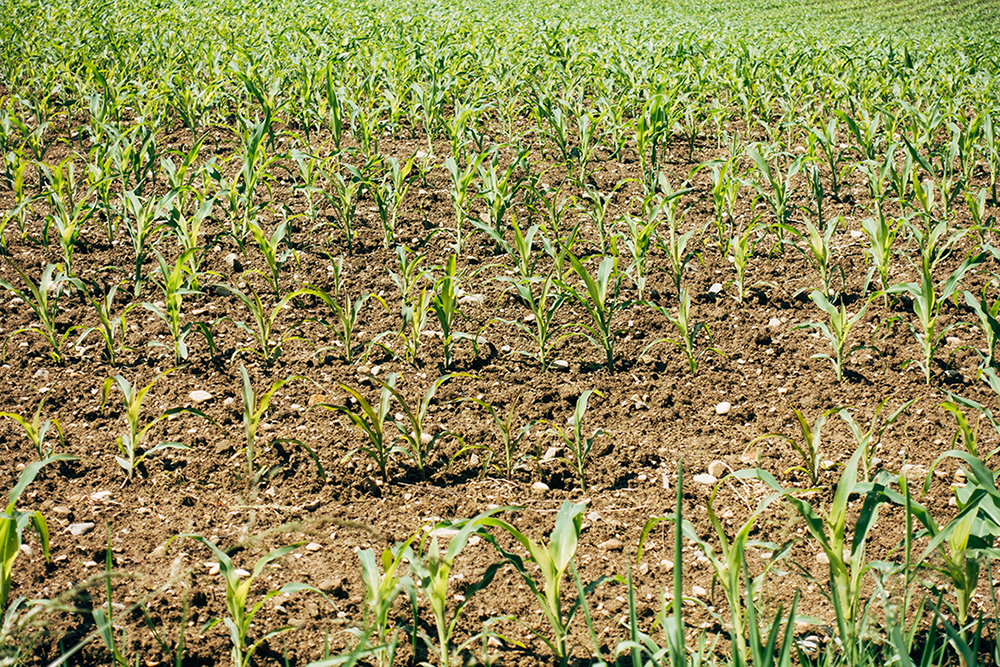
Nigeria’s Changing Fertilizer Sector (Part 1)
In the past decade, there has been over $7 billion worth of private-sector investments into Nigeria’s agriculture sector. Why, and what is the impact of these investments? What does it mean for Nigerian farmers? In this blog, we explore these and other changes happening in the Nigerian fertilizer market.
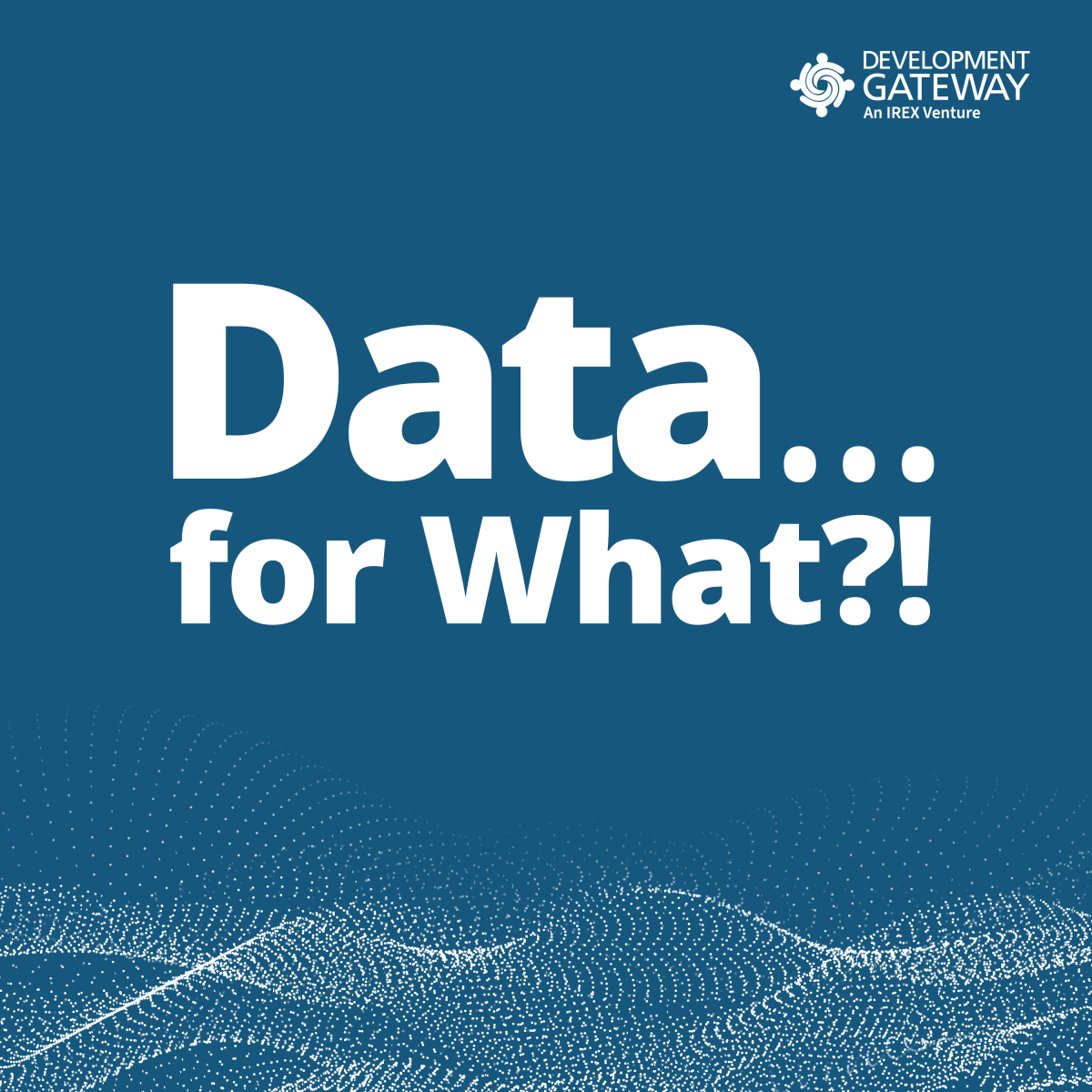
Geography: Expanding & Deepening DG’s Regional Footprint
In Episode 3 of "Data… for What?!", Development Gateway’s new podcast, we explore how we are prioritizing our geographical focus in the coming years. Conversations with Vanessa Baudin Sanchez, Carmen Cañas, and Charlene Migwe-Kagume highlighted the opportunities and challenges of expanding our portfolio in Central America and deepening our work and partnerships in West, East, and Southern Africa.
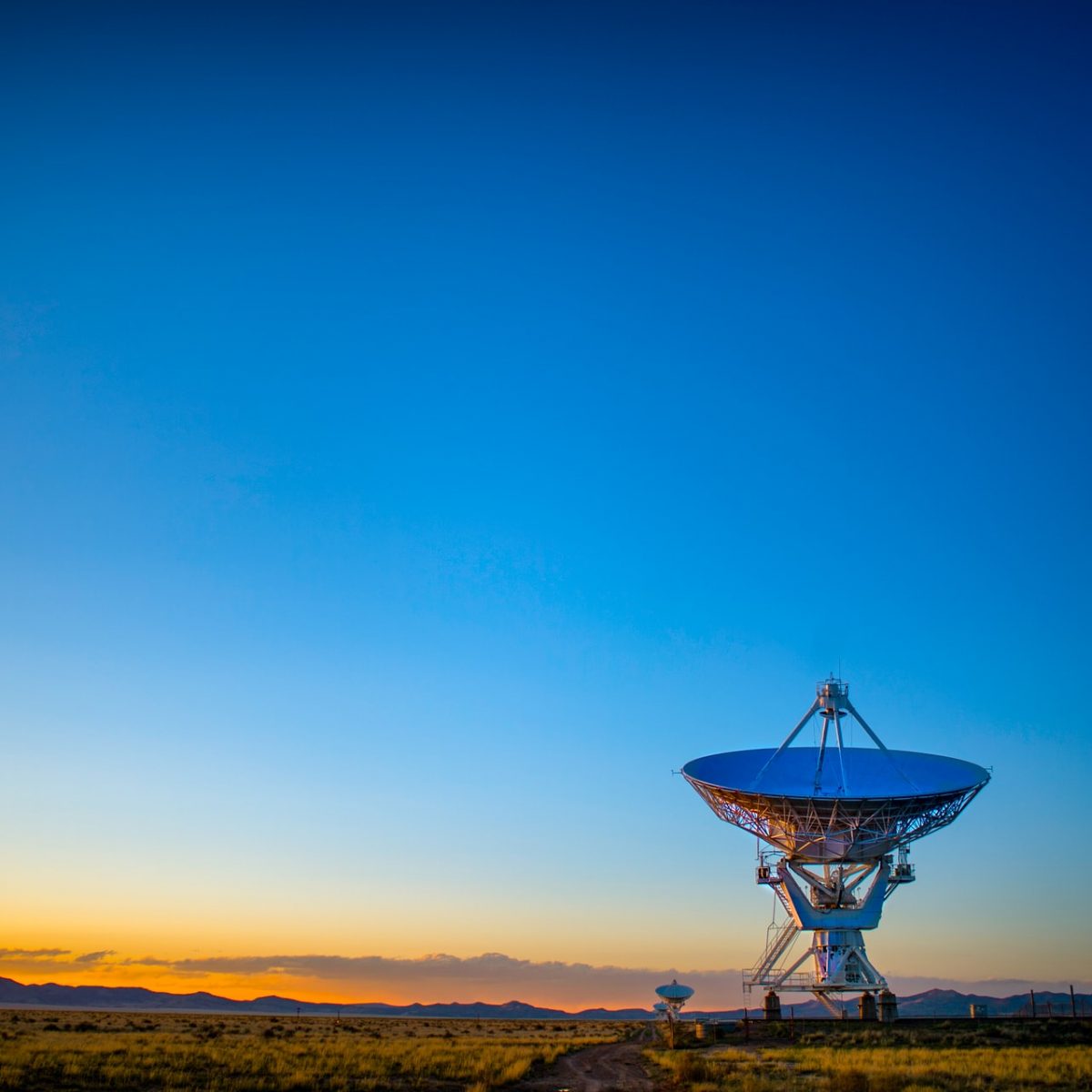
What We Know So Far: Best Practices in Developing Data Governance Frameworks
Data governance frameworks are essential as data and technology continue to become increasingly central to how governments, institutions, groups, and individuals all interact with one another and among themselves. Here are the best practices DG has learned in creating data governance frameworks so far.
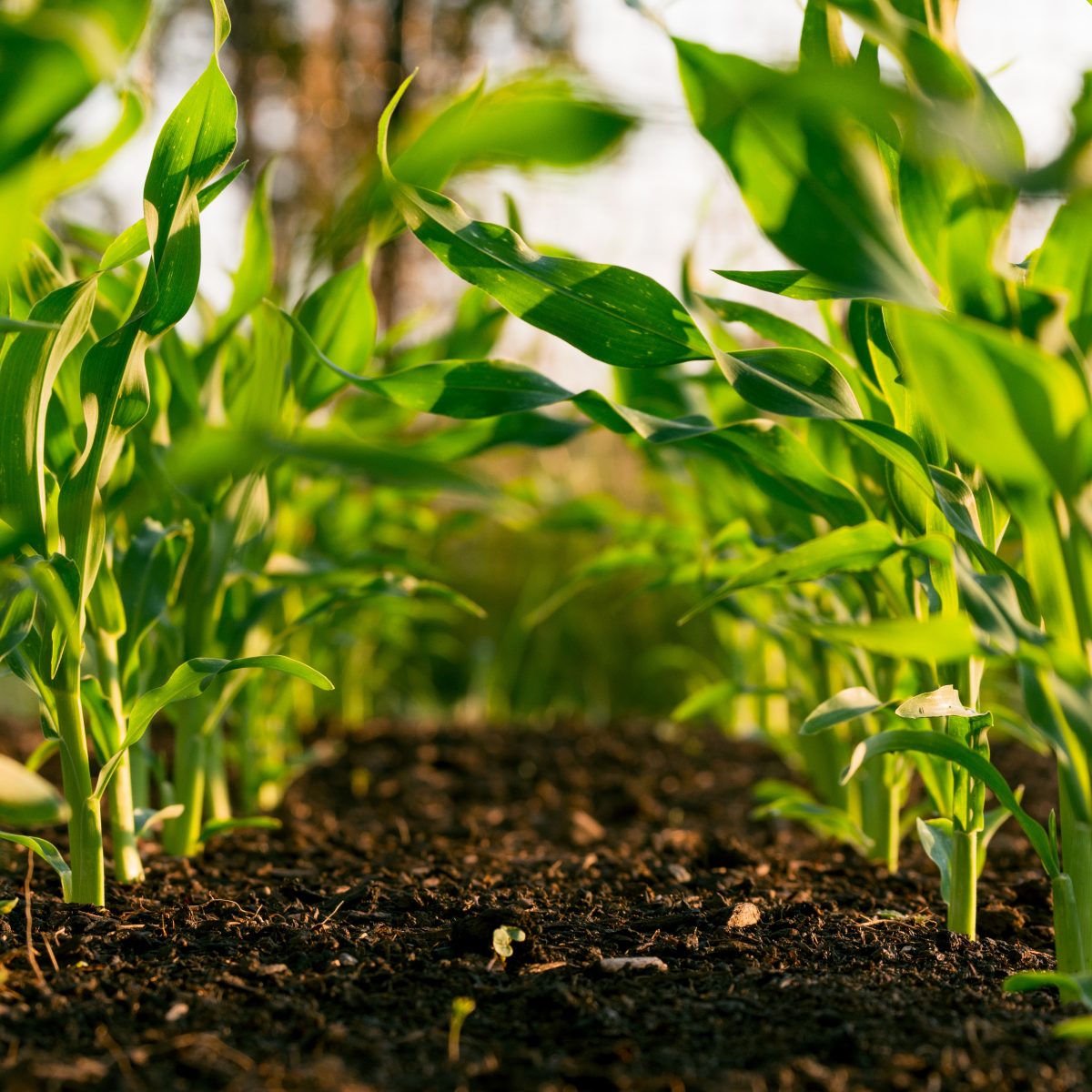
Price & Availability: the Impact of Russia’s Invasion of Ukraine on African Fertilizer Markets
In response to fertilizer availability challenges in sub-Saharan Africa which are fueled by the ongoing Russian invasion of Ukraine along with a host of pre-existing factors including COVID-19, VIFAA is now launching the Africa Fertilizer Watch to track regional and country-level market indicators, productivity and production distortions on food production and agriculture.
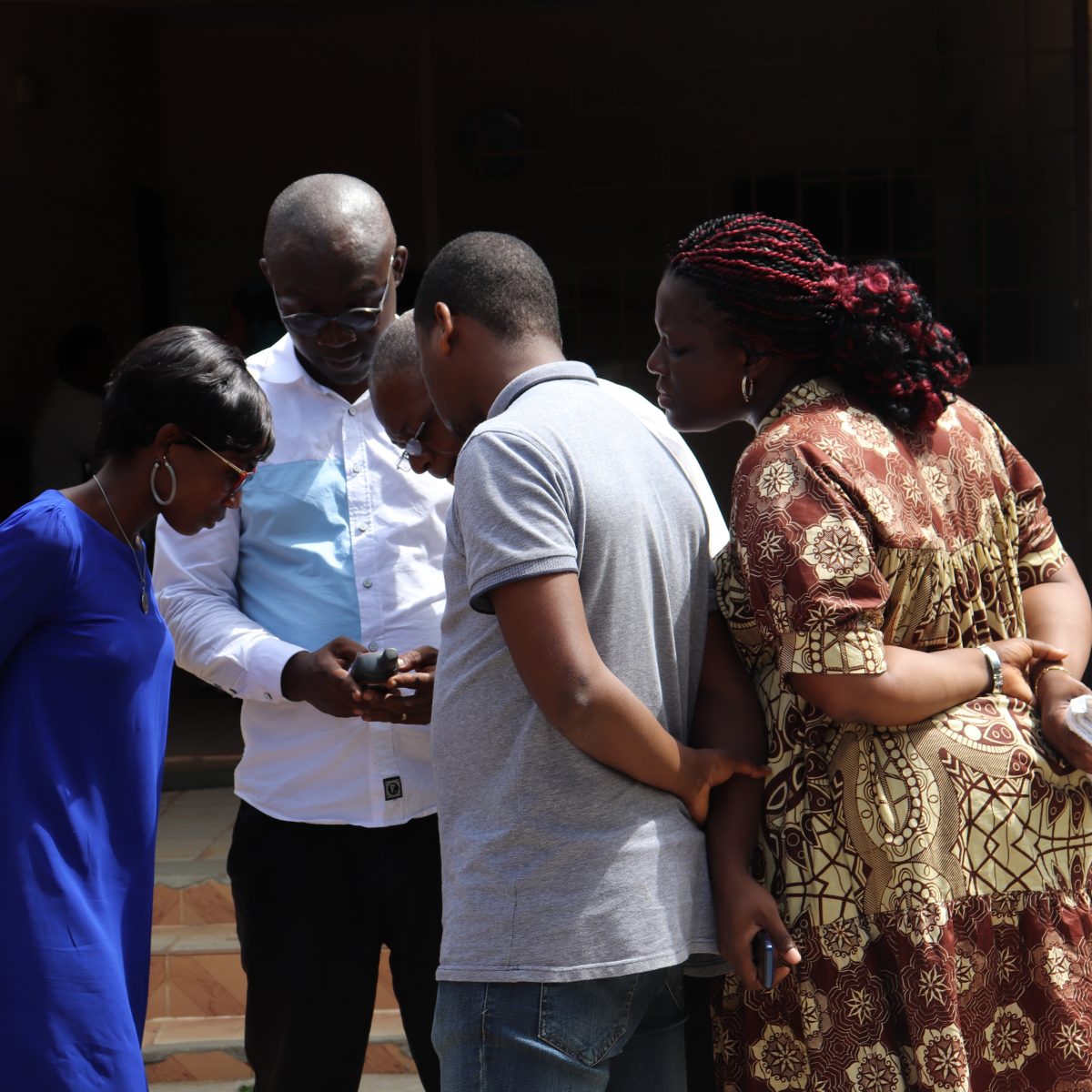
How IREX and DG’s Data Ecosystem Assessment Tools Advance Local Priorities
IREX’s Data Compass and Development Gateway’s CALM are methodologies for assessing data ecosystems. While each methodology produces different outputs based on different needs, both prioritize local collaboration and development to produce insights and outputs that reflect local priorities and actionable recommendations that institutions can own and implement.
Cashew-IN Platform Demo
Development Gateway (DG) and Cultivating New Frontiers in Agriculture (CNFA) have partnered to launch an innovative platform to support the West African cashew sector and increase profitability for cashew farmers. Comprehensive and reliable data is required for cashew farmers, producers, processors, and others in the West African cashew sector to increase profits, reduce loss, and improve global competitiveness. In this video, Vanessa Baudin Sanchez and Nadejda Mândrescu demonstrate the features of the Cashew-IN platform and discuss the underlying technology.
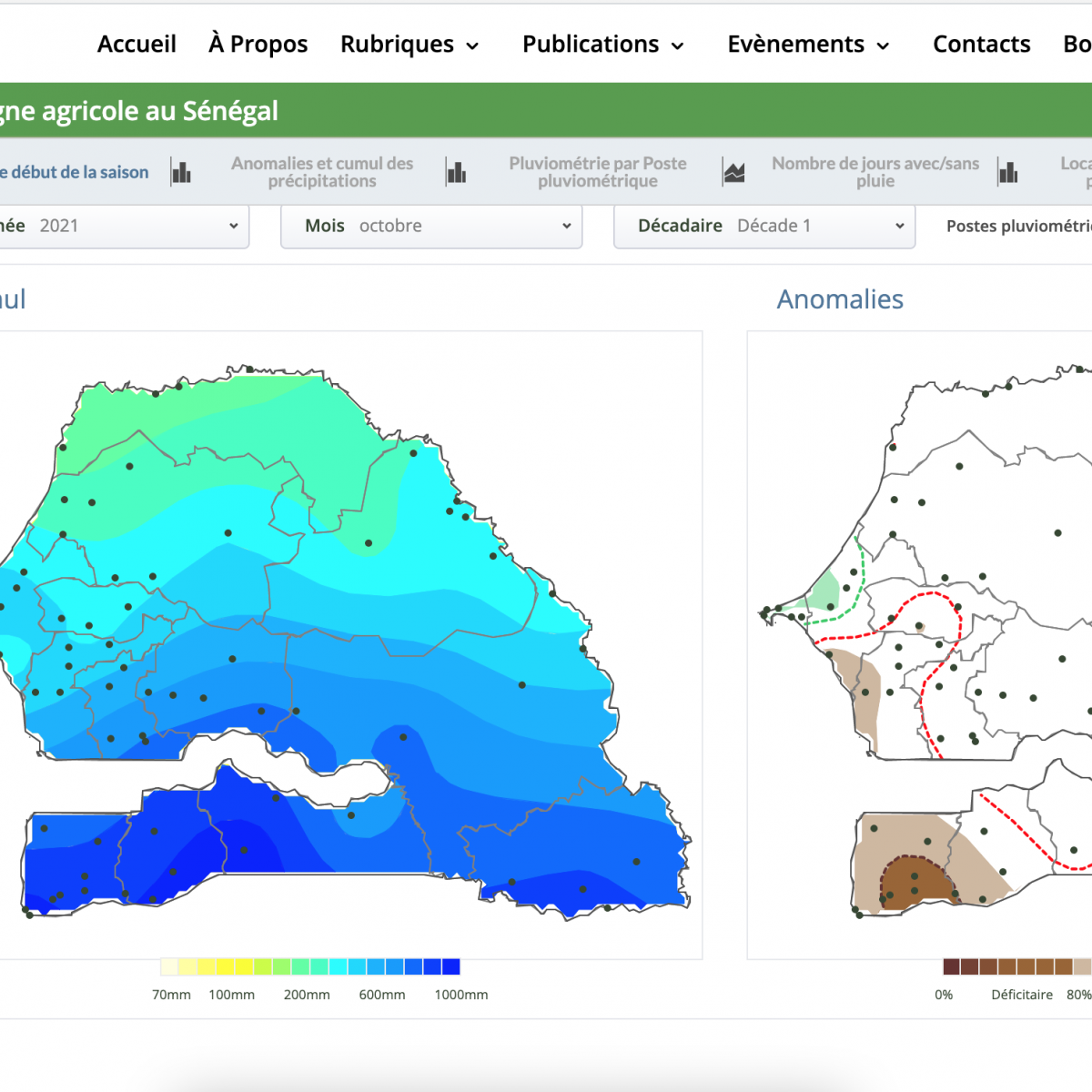
AD3ventures in Mapping
Administrative data – data from civil registration and vital statistics systems, and sectoral (health, education, judicial, social protection) management information systems – is rich with insights on citizen needs and priorities. Though timely and disaggregated administrative data can complement official statistics, it comes with its own data quality, availability, and use challenges.
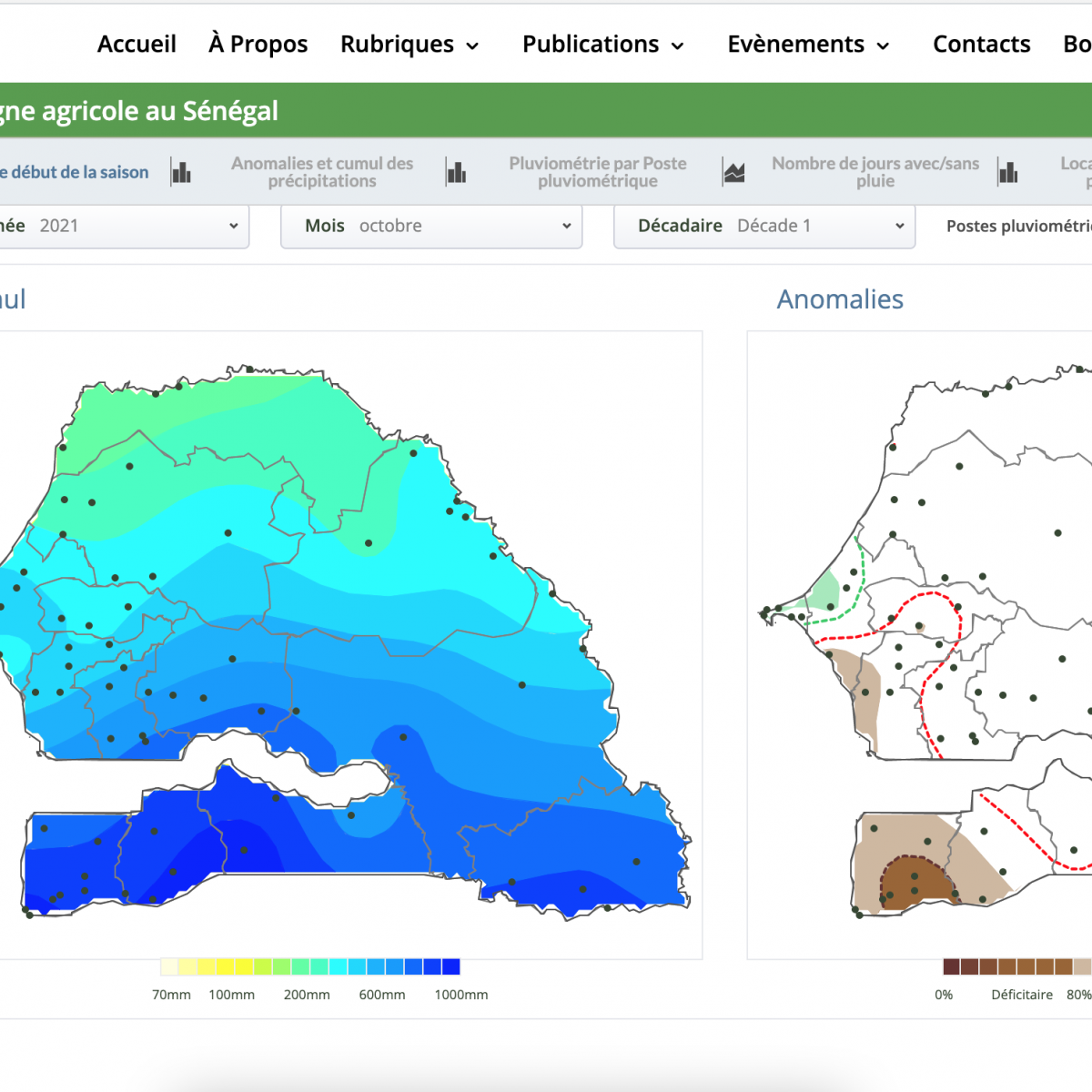
AD3 ou une aventure cartographique
Par le biais d'AD3, nous avons développé un tableau de bord qui se concentre sur le climat, les prix du marché, les maladies du bétail, le niveau des fleuves et les précipitations annuelles, entre autres thèmes. Si le tableau de bord lui-même est un référentiel d'informations utile, la méthode d’élaboration des cartes et d’itération des commentaires des utilisateurs a permis d'identifier les bonnes pratiques en matière de visualisation des données.
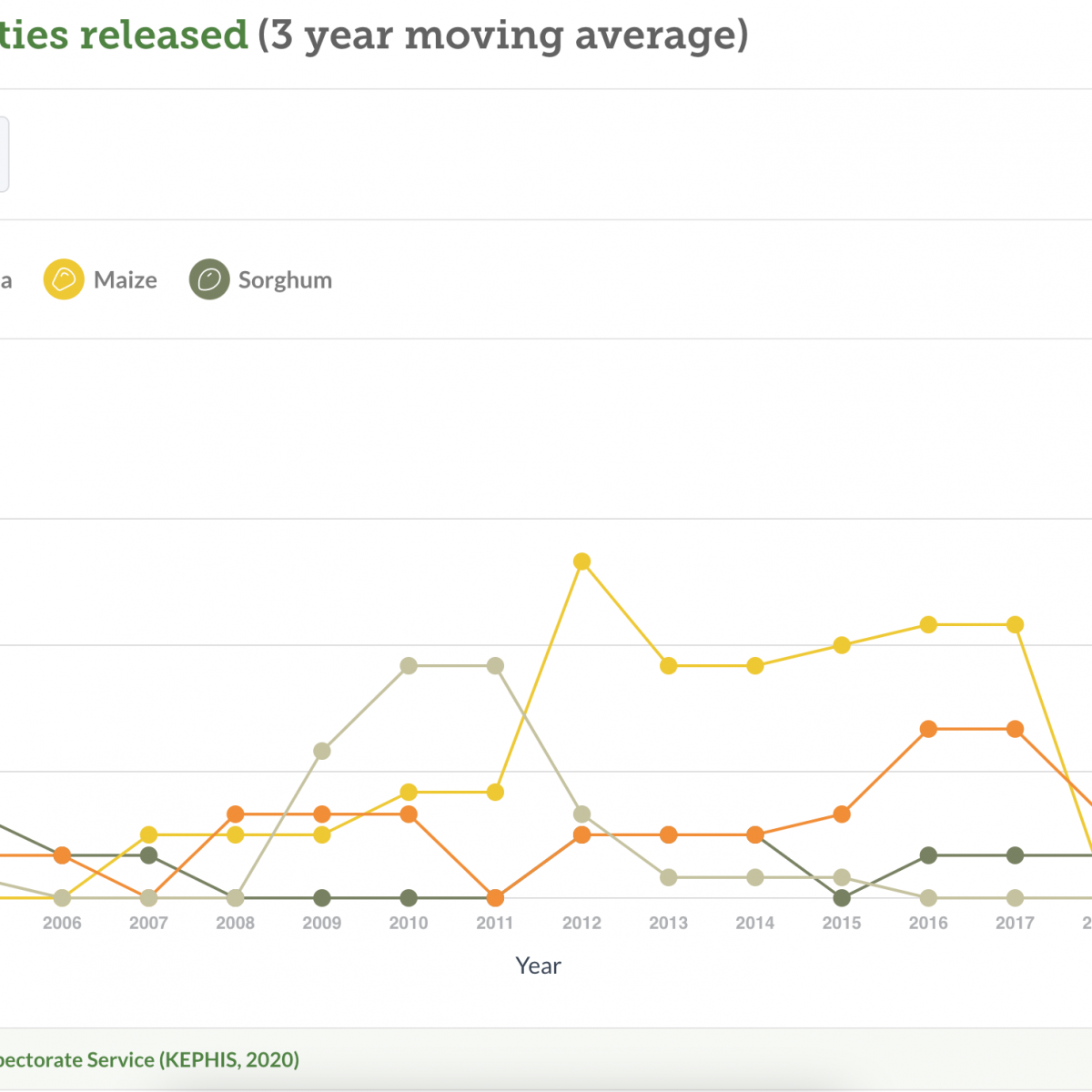
Designing Data Visualizations: Merging Best Practices and Design Thinking
DG has been co-designing data visualizations with partners and stakeholders for over a decade. Thinking about the ways people process information is crucial to developing easy-to-understand data visualizations. In this post, we examine best practices for incorporating user-centered design into our data visualization outputs.
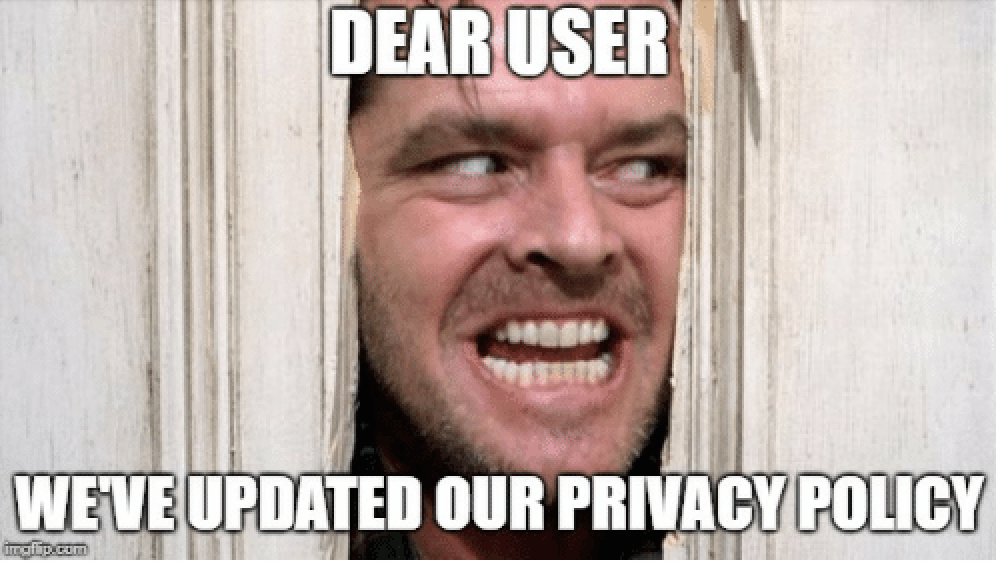
All In Your Business: Talking Data Governance and Privacy at Development Gateway
Our work is at the intersection of open data, technological development, and strategic advising to improve data use. We see growing questions about how we as a global community manage, share, reuse, and store data that is integral to our existing and future work. This blog is the start of a conversation we want to have.